
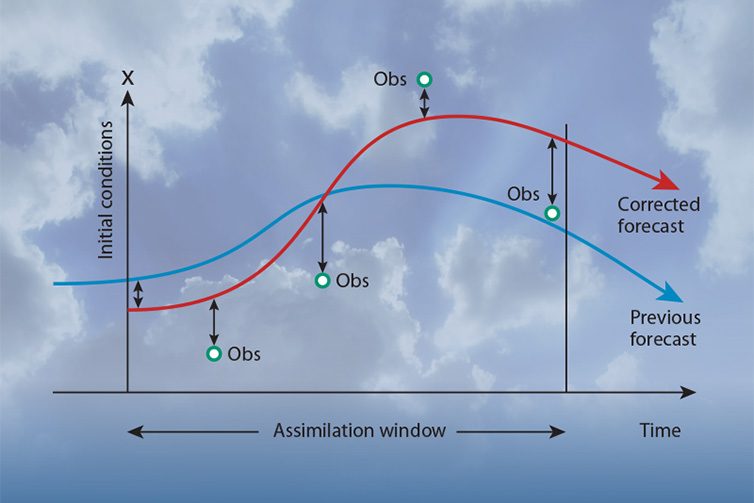
Twenty-five years ago, ECMWF introduced ‘4D-Var’ to the assimilation of observational data into its weather model. This four-dimensional variational data assimilation technique is used to establish the initial conditions of forecasts.
To do so, it adjusts a short-term forecast slightly so that it better fits the weather observations made over a short period of time, called the assimilation window.
4D-Var has led to substantial improvements in the Centre’s forecasts. A summary of how it works can be found in a previous article marking 20 years of 4D-Var. A more detailed overview is given in the journal Weather.
Over the last few years, ECMWF scientists have worked on some changes to 4D-Var. To mark the 25th anniversary, we focus here on the growing use of machine learning in data assimilation.
Handling model bias
In traditional ‘strong-constraint’ 4D-Var, it is assumed that the model is perfect. In ‘weak-constraint’ 4D-Var it is recognised that it is not, and some adjustments are made.
An example of this is adjustments to temperature in the stratosphere. Here, ECMWF’s Integrated Forecasting System (IFS) is known to produce large biases. These biases are also present in the short-term forecasts used in data assimilation.
To address this, weak-constraint 4D-Var changes not just the initial state of a forecast to bring the forecast trajectory closer to observations. It also adds an additional term η to the model to account for bias in the forecast.
It does so by accounting for the forecast bias throughout the assimilation window.
In data assimilation, a previous forecast, here called ‘first-guess trajectory’, is replaced by a corrected forecast, called the ‘analysis trajectory’. Weak-constraint 4D-Var applies changes η to the analysis trajectory to remove bias from it as far as possible.
“In weak-constraint 4D-Var, the model trajectory is adjusted according to what observations tell us about systematic errors in the model,” says ECMWF’s Massimo Bonavita.
At the moment, weak-constraint 4D-Var is only implemented in the stratosphere because here systematic model errors are relatively big. Adjustments in the troposphere are being worked on but are trickier, because here systematic model errors are much smaller.
Weak-constraint 4D-Var also requires bias-free or, more commonly, bias-corrected observations, which is not always the case in the troposphere. “We are working on it though to get it right,” Massimo says.
According to Massimo, the adjustment of the forecast trajectory in weak-constraint 4D-Var can be seen as an example of machine learning. “This is because it doesn’t work to construct a new physical model but to correct the current model with data-driven information.”
Today, research is also progressing on the use of a more conventional type of machine learning in 4D-Var data assimilation, which is based on the use of neural networks.
Correcting model error through machine learning
Scientists are striving to use machine learning that uses neural networks to come up with hybrid models able to estimate and correct model error.
“A neural network is trained to learn model error, and we can potentially apply this neural network not just to the short-term forecasts used in data assimilation, but all the way up to climate forecasts,” Massimo says.
A recent machine learning workshop which ECMWF organised jointly with the European Space Agency (ESA) gives an idea of the wide range of potential applications.
In data assimilation, one of the ideas is to use a neural network to vary the static η that is added to the analysis trajectory in weak-constraint 4D-Var.
Factors that determine how it is varied include the current forecast state, time of day, month, and lat/lon coordinates. The neural network estimates model errors on this basis.
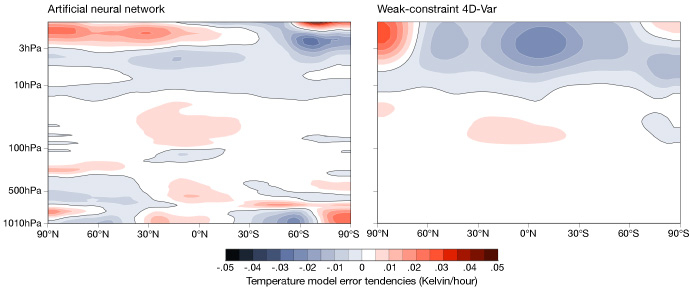
The charts show temperature model error tendencies from about 1 hPa to 1010 hPa estimated by an artificial neural network (ANN) and by weak-constraint 4D-Var. Values are averaged over a one-week period starting on 20 July 2019. Weak-constraint 4D-Var is restricted to the stratosphere and therefore shows no tendencies below. Adapted from: M. Bonavita and P. Laloyaux, 2020, JAMES, doi:10.1029/2020MS002232 (CC BY 4.0).
“Essentially you apply statistical corrections based on all these inputs,” Massimo observes. “A fair way of putting it is that we combine a physical model with a statistical model and end up with a hybrid model.”
The future, then, might lie in physical models having a statistical component in the form of neural networks.
“It’s another form of information that we can bring to bear on data assimilation and forecasting. In five or ten years’ time, we may be finding that machine learning is present everywhere in the forecasting process,” Massimo says.
“The basic structure of 4D-Var is very sound on the other hand: it’s the right probabilistic approach. While the concept of 4D-Var will survive, machine learning tools will increasingly be used as part of it.”