
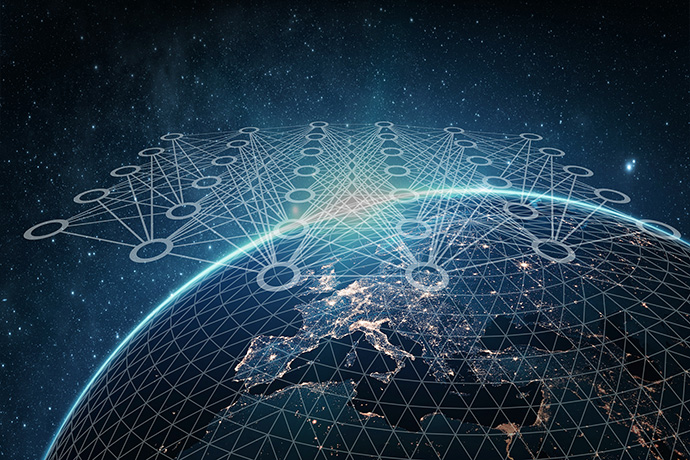
The European Space Agency (ESA) and ECMWF held an online workshop on Machine Learning for Earth System Observation and Prediction (ML4ESOP) from 15 to 18 November 2021.
The annual event attracted over 1,100 participants from 85 countries. They heard more than 30 talks on the latest research into the application of machine learning (ML) to Earth system monitoring and predictive modelling.
Highlights particularly relevant to weather prediction included talks on the use of machine learning in Earth system data assimilation, in geophysical forecasts, in evaluating satellite observations, and in post-processing and dissemination.
ECMWF Director of Research Andy Brown said: "There are both huge opportunities and huge challenges in observation processing, data assimilation and modelling as part of Earth system observation and prediction, and machine learning will undoubtedly play a significant part in meeting those challenges. This workshop, convened jointly by ESA and ECMWF, has been a great opportunity to bring together a broad community to explore the state of the art and the road ahead, and I’ve been extremely impressed by the range and depth of material covered."
Earth system data assimilation
A common theme in the talks presented in this area was the increasing convergence and blending of ML technologies with data assimilation methodologies long established in the Earth sciences.
Examples of this synergy were given in talks by Sibo Cheng and Daisuke Hotta, where it was shown how to perform data assimilation in an optimal latent space, which is discovered with ML techniques.
Another prominent theme has been the use of ML methods to diagnose and correct model errors in a data assimilation framework, both in simplified models (Alban Farchi) and in state-of-the-art forecast models (Marcin Chrust).
An emerging area is the application of neural networks to model the links between observations and the forecast model in situations where a physical model is unavailable or highly uncertain. Progress in this direction was discussed by Alan Geer, Sean Healy and others.
Sean Healy’s talk was entitled ‘Towards the Direct Assimilation of Scatterometer Backscatter Triplet’.
Hybrid geophysical forecasting
There is growing interest in using ML solutions for drastically increasing the efficiency of high-resolution geophysical models and for climate applications (see for example the talks by Tom Beucler and Janni Yuval).
Efforts to improve current models through ML, and substituting them completely when the underlying physics is complex and not completely understood, were presented.
While most of the talks were aimed at NWP and climate prediction, the application domain is in fact much larger and covers for example areas like water-resources management (Stefano Bagli) and wildfire forecasting (Sibo Cheng).
Sibo Cheng gave a presentation on ‘Data-Driven Surrogate Model with Latent Data assimilation for Wildfire Forecasting’.
Better satellite data products
One area of great interest is the application of ML technologies to extract more complete and meaningful information from the huge amount of raw satellite observations of the Earth system that are currently available.
This includes new takes on established activities, like geophysical retrievals (see for example the presentations by Mario Echeverri Bautista and François-Marie Bréon), and also more recent applications inspired by ML successes in areas like super-resolution (Diego Valsesia), features extractions (Georgios Balasis), and others.
Diego Valsesia gave a talk on ‘Permutation invariance and uncertainty in multitemporal image super-resolution’.
From the working group discussion, it is clear that ML techniques will be increasingly used due to their computational efficiency and the possibility to apply them to problems where the physical modelling is not sufficiently mature.
Post-processing and dissemination
Post-processing of numerical forecasts and dissemination of user-tailored products are two application areas that are very suited to ML techniques.
A number of talks and posters discussed ML solutions to improve the calibration of numerical output (John Bjørnar Bremnes, Tobias Finn, Tom Hengl). They consistently showed improved performance over more traditional statistical methods.
The dissemination aspect was also extensively covered. Talks ranged from using ML to downscale air pollution estimates obtained by the fusion of different information sources to the identification of flooded areas using Sentinel-1 SAR imagery with semi-supervised learning (Siddha Ganju) and supporting agricultural monitoring and food production.
Siddha Ganju talked about ‘Citizen Scientists Tackling Devastating Floods and Disaster Relief with Semi-supervised Deep Learning’.
Working groups and e-poster event
The final day was dedicated to working groups which discussed current limitations and ways forward in machine learning for Earth system work. The findings were summarised at the closing plenary session.
The workshop was also accompanied by an e-poster side event with some 30 presentations from academia, research institutes and industry.
“The number of participants and the quality of the oral and poster presentations have clearly made this workshop a very successful one,” said ECMWF scientist Massimo Bonavita.
“I’ve been particularly impressed by the breadth of the proposed ML applications and their maturity, showing that ML can be a significant addition to the NWP and climate prediction value chain. This is for us a great encouragement to continue this series of ML workshops next year, when we will hopefully be able to host participants in person at ECMWF in Reading, UK!”